Like every world-changing technology, AI has raised a slew of concerns ranging from the existential to the practical. As an example, while AI doomers are sounding the alarm on the impending AI apocalypse, business leaders are more concerned about AI leaking sensitive data.
No matter where you fall on the spectrum of AI anxiety, most of us want our AI systems to operate safely and ethically. This is where responsible AI comes in. Responsible AI has been defined in different ways by different organizations (see definitions from Microsoft, Google, and Meta), but at its core focuses on how to mitigate risk and promote security, privacy, and fairness in the development and deployment of AI systems.
Every organization building AI-powered products should be aware of responsible AI. While the largest organizations have dedicated teams, most organizations will need to adopt new practices and tooling to deliver AI products responsibly. In this blog we’ll discuss what responsible AI practices we expect companies to adopt and the opportunities we see for new tooling.
What are the pillars of responsible AI?
Responsible AI is an umbrella term for all ethical, safety, and governance concerns around AI. While responsible AI incorporates concepts like transparency, explainability, and control, most responsible AI activity is in service of three thematic pillars: security, privacy, and fairness.
- Security: AI systems should be robust and resistant to malicious attacks and tampering that seek to compromise or alter the system’s functioning in any way, or exfiltrate sensitive information such as the system’s training data, sensitive user or business data, or details about the system itself.
- Privacy: AI systems should maintain individual privacy and prevent misuse or exposure of personal data from their training set or collected from users.
- Fairness: AI systems should treat all people fairly and be carefully evaluated to detect and eliminate bias. AI systems shouldn’t discriminate against any group of people in the decisions they make or the content they generate.
AI safety is a concept that comes up frequently in discussions of responsible AI. Safety is generally concerned with ensuring that AI systems behave as intended. There is substantial overlap between safety and security, so we did not call it out as a separate pillar.
Regulations play a role in how organizations will prioritize and adopt responsible AI solutions. AI systems need security and privacy safeguards to ensure compliance with data protection regulations like GDPR and CCPA/CPRA. Systems need to be evaluated for fairness in order to comply with NYC’s AI Bias law, which applies to companies using AI for employment-related decision making, e.g. recruiting or HR. This is also important for compliance with existing anti-discrimination laws as well as proposed legislation targeting algorithmic bias in industries like insurance and banking. The EU AI Act was recently passed by the EU Parliament and more AI-specific regulation is expected to follow.
What tools will help organizations working toward responsible AI?
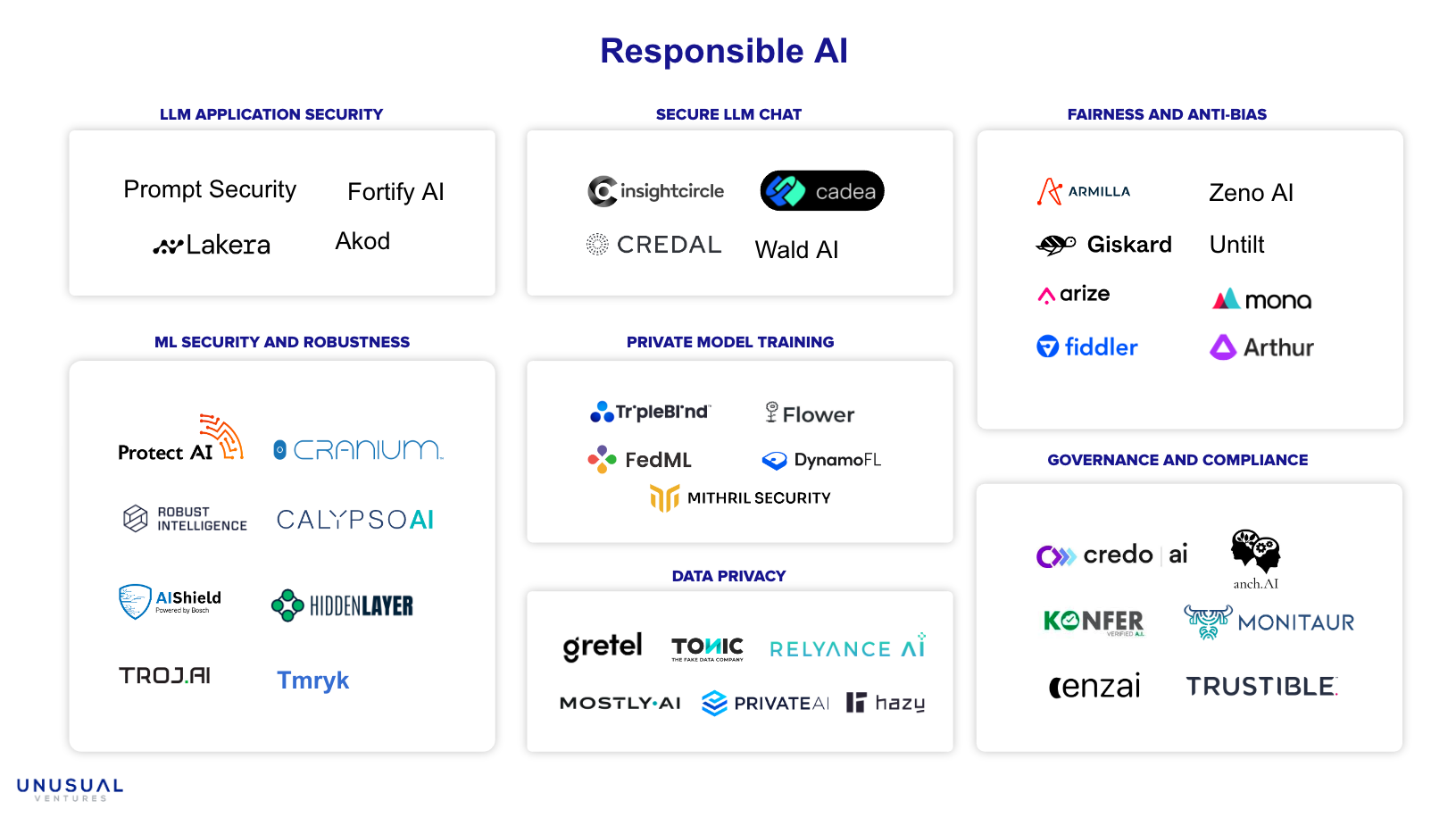
A new landscape of tools is emerging to help organizations develop and deploy AI systems responsibly. On the development side, we’re seeing tools for preparation and handling of training data, and for the evaluation and testing of models pre-deployment. In production, we are seeing tools for monitoring models and detecting non-compliant or anomalous behavior, as well as tools for governance and compliance of AI systems.
Specialized tooling is needed to address core requirements of each of the three pillars: security, privacy, and fairness.
Security tools for AI
There are security risks across the AI lifecycle, from development to deployment. ProtectAI focuses on helping organizations secure their ML supply chains and protect against threats in open-source ML libraries and ML development tooling. Robust Intelligence, Calypso, Troj.ai, and Tmryk focus on testing and evaluating AI models for robustness against known adversarial threats. HiddenLayer, Cranium, and AIShield focus on monitoring AI infra to detect and respond to adversarial attacks.
The rise of LLMs has led to distinct security concerns that warrant dedicated solutions. For LLM app developers, prompt injection attacks pose AI security and safety concerns. Fortify AI, Prompt Security, Akod, and Lakera focus on solving the specific security challenges of deployed LLM apps. For enterprise users of LLM apps, data protection is a concern that spans both security and privacy. Credal, Insightcircle, Wald AI, PrivateAI, and Cadea focus on enabling secure enterprise adoption of LLM chat.
Privacy tools for AI
Most privacy risk starts with the training data. To address this, tools from companies such as Gretel, Tonic, Mostly AI, and Hazy can generate synthetic datasets that preserve the characteristics of the original data. When synthetic data isn’t an option, privacy-preserving machine learning techniques enable training on sensitive data while ensuring privacy and compliance. Mithril security leverages confidential computing environments to train models on sensitive data, while FedML, Flower, and DynamoFL enable training on disparate data sets via federated learning.
Fairness and anti-bias tools for AI
Pre-deployment model testing and evaluation is necessary to ensure that AI models are not biased. New startups Untilt and Zeno AI are working on this problem. Continuous monitoring is also needed to monitor production models for bias creep. Arize, Mona, Fiddler, and Arthur are tackling this problem as part of their broader observability solutions.
Governance and compliance tools for AI
Existing and emerging regulation means that tools are needed for GRC teams to manage governance and compliance of AI systems. Startups Enz.ai, Monitaur, Credo, Anch.ai, and Konfer are building in this space.
Predictions for responsible AI
- Organizations will adopt products across multiple responsible AI categories.
Adoption will be driven by different personas and roles. Security teams will need tools to evaluate and monitor AI systems from a security perspective; product teams will require different tools to evaluate and monitor AI systems for fairness and anti-bias.
- Specific tooling for LLMs will continue to emerge.
The non-deterministic nature of large language models (LLMs) results in some unique requirements when it comes to responsible AI. We believe that new tooling will emerge specifically for LLM testing and evaluation, observability and monitoring, and security.
- Regulation will drive urgency to adopt responsible AI solutions.
Both existing and upcoming regulation will drive urgency for enterprises to adopt responsible AI tooling across all categories.
We are excited to meet with folks working on responsible AI within their organizations or building new products. Don’t hesitate to reach out on Linkedin or at allison@unusual.vc. We’d love to hear your perspective.
Lorem ipsum dolor sit amet, consectetur adipiscing elit. Suspendisse varius enim in eros elementum tristique. Duis cursus, mi quis viverra ornare, eros dolor interdum nulla, ut commodo diam libero vitae erat. Aenean faucibus nibh et justo cursus id rutrum lorem imperdiet. Nunc ut sem vitae risus tristique posuere.