Three Common Pitfalls of AI Pitches
Given the explosion of interest from founders and investors alike, it’s unsurprising that AI-related companies raised a total of $16.9B in 2019. That being said, I know some founders still struggle to get off the ground. Unfortunately, it takes more than just a great idea and a high-performing machine learning model to raise capital and build a lasting company.
In Part 1 of this post, I’ll detail some of the common pitfalls we see seed-stage founders make when pitching their AI startups. (This comes from my own experience from hearing hundreds of AI pitches.) I share these observations to give an inside look into the black box (excuse the pun) of fundraising from VCs. Hopefully this enables founders to improve their pitch and increase their likelihood of success. Of course, it’s worth noting that the recommendations below are my own observations and there are exceptions to every rule. In Part 2, I talk about some of the areas within the AI space we’re excited about at Unusual and where we see big opportunities.
Pitfall #1: Believing your algorithm is your competitive advantage
Many startups think that their algorithms are their competitive advantage. They may in fact have better algorithms, but that’s typically not a differentiated moat. It’s easy enough today to import scikit-learn or use Keras or TensorFlow. While expertise is required for feature generation and sophisticated custom algorithms can provide value, it is still not nearly as important as something that’s often overlooked--the developer experience.
Unusual Partner Andy Johns, has a great anecdote from his time as President at Wealthfront. There were other robo-advisory firms at the time that claimed they had the highest returns or lowest cost, but it was Wealthfront and Betterment that carved out substantial early market share because they specifically did NOT position themselves as having the best algorithms. Instead, they positioned themselves as leveraging automation to create the most convenient and transparent user experience. Their focus on ease-of-use, or more specifically the user as a whole, was what led them to succeed in the market. The same applies to the AI space.
Another example comes from Unusual Partner Jyoti Bansal. In the early days of AppDynamics, the team posted signs around the office of “2 minutes or less” to underscore the need to get new AppDynamics users up and running with the product within that time frame. While the quality of the product and its insights were certainly important, even more paramount was the seamlessness in the developer experience and getting potential customers to an “aha moment” as quickly as possible. Even if AppDynamics had the best performance monitoring solution, it would not have been nearly as successful as it was if it were difficult for users to instrument and adapt AppDynamics to their applications.
While Wealthfront and AppDynamics were not necessarily AI-first companies, the general importance of user experience is something we see across industries, and in particular for developer tooling companies like ML tools. ML companies like Hugging Face have focused squarely on developer experience and adoption rather than monetization, and have experienced exponential growth as a result. Algorithms are now a commodity. The new reality is that developer experience is king and should be top of mind in every AI pitch.
Pitfall #2: Boiling the ocean with an “End-to-end ML” business model
Several companies like C3.ai, Palantir, and Landing AI are industry-agnostic and attempt to provide end-to-end ML. Each of these companies have strong platforms (full disclosure: I worked at Palantir and C3.ai). However, not only is creating a single platform that provides end-to-end ML capabilities incredibly difficult, but more often than not, it requires a ton of consulting work, extensive time with clients to generate domain-specific features, and time spent developing integrations with unique systems across industries. The result is a costly business model and suboptimal ML models.
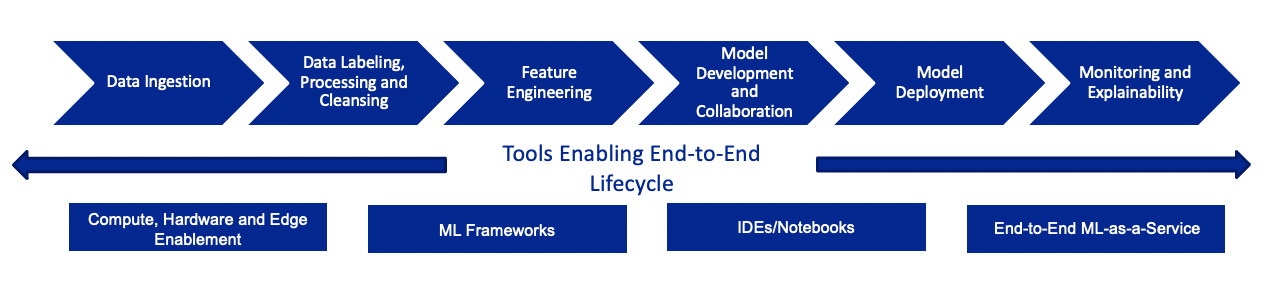
The ML infrastructure space will inevitably consolidate into providers that are more able to provide end-to-end ML capabilities, but one of the most important things early-stage investors (particularly Seed/Series A) look for is focus. A company should have a vision for the future and how their product can become a billion-dollar opportunity by expanding to adjacent areas of the model lifecycle, but they need to have a clearly defined beachhead. Startups that promise an end-to-end ML solution often lack that wedge of the market they can tackle and specialize in. A horizontal solution works well when tackling a specific component of the ML pipeline (e.g., DataRobot for AutoML; Databricks for compute), but otherwise vertical solutions can integrate far more easily with a subset of important solutions and create highly specialized and optimized models.
Pitfall #3: Assuming that because you built a great product, people will use it.
You can have the best product in the world, but without a proper Go-To-Market Strategy (GTM), it may never see the light of day. This is the one area that I see entrepreneurs (AI startups or otherwise) underemphasize when pitching investors. Do you want to use a bottom-up adoption approach (e.g., getting developers to use it in a company), middle-out, or a top-down approach (e.g., selling to a VP of Engineering)? If you do bottom-up, how do you plan on successfully promoting developer evangelism (content strategy, open source distribution, freemium, etc.)? If top-down, what will the sales cycle look like and how many sales reps will you need? What is the ideal customer persona (ICP) you are targeting (their role, the size of the company, the industry, the tech stacks they are using, how technically sophisticated these customers are, etc.)? Do you have a “big splash” press launch strategy or will you get 5-10 design partners to iterate on the product with you before launching publicly?
VCs look for two things: 1) a large enough market such that the startup can have a $1B exit, or a smaller market that is growing rapidly with opportune timing to capitalize on said growth; and 2) a proper GTM strategy to capture a beachhead to position the company to grow exponentially.
At Unusual, we’ve observed that software is no longer sold, it’s adopted. Thus, we tend to strongly prefer bottom-up adoption GTM. For AI startups, this typically means an open source, freemium, or free trial component to the product. We’ll have another post on the benefits of such an approach, but the more your product can become ubiquitous and the go-to-tool for X, the better.
Closing Thoughts
Believing your algorithm is your competitive advantage, attempting to build an end-to-end ML solution instead of focusing with a beachhead wedge, and neglecting a bottom-up motion are common pitfalls we see in AI pitches. In contrast, the best AI pitches we’ve seen understand the utmost importance of an excellent developer experience, have a clearly-defined beachhead, and have developer evangelism built into their product and GTM strategy.
Of course, there are exceptions to the rule and successful companies that buck the pitfalls described. Still, it is important for founders to think through these considerations as they ready themselves to pitch to investors and start their companies.
Lorem ipsum dolor sit amet, consectetur adipiscing elit. Suspendisse varius enim in eros elementum tristique. Duis cursus, mi quis viverra ornare, eros dolor interdum nulla, ut commodo diam libero vitae erat. Aenean faucibus nibh et justo cursus id rutrum lorem imperdiet. Nunc ut sem vitae risus tristique posuere.